How Smart Is AI Video Analytics (And Will It Ever Be Really Intelligent)?
Video surveillance is commonly associated with security. But in most cases, it's used to record incidents and assist in investigations after the fact rather than prevent undesirable events. Artificial intelligence–powered video analytics is a highly promising trend that fundamentally changes the way things work. Extracting manageable data from a video stream can help recognize risky situations early on, minimizing damage and, ideally, completely avoiding emergencies. At the same time, AI significantly expands the areas of application of video surveillance beyond security systems.
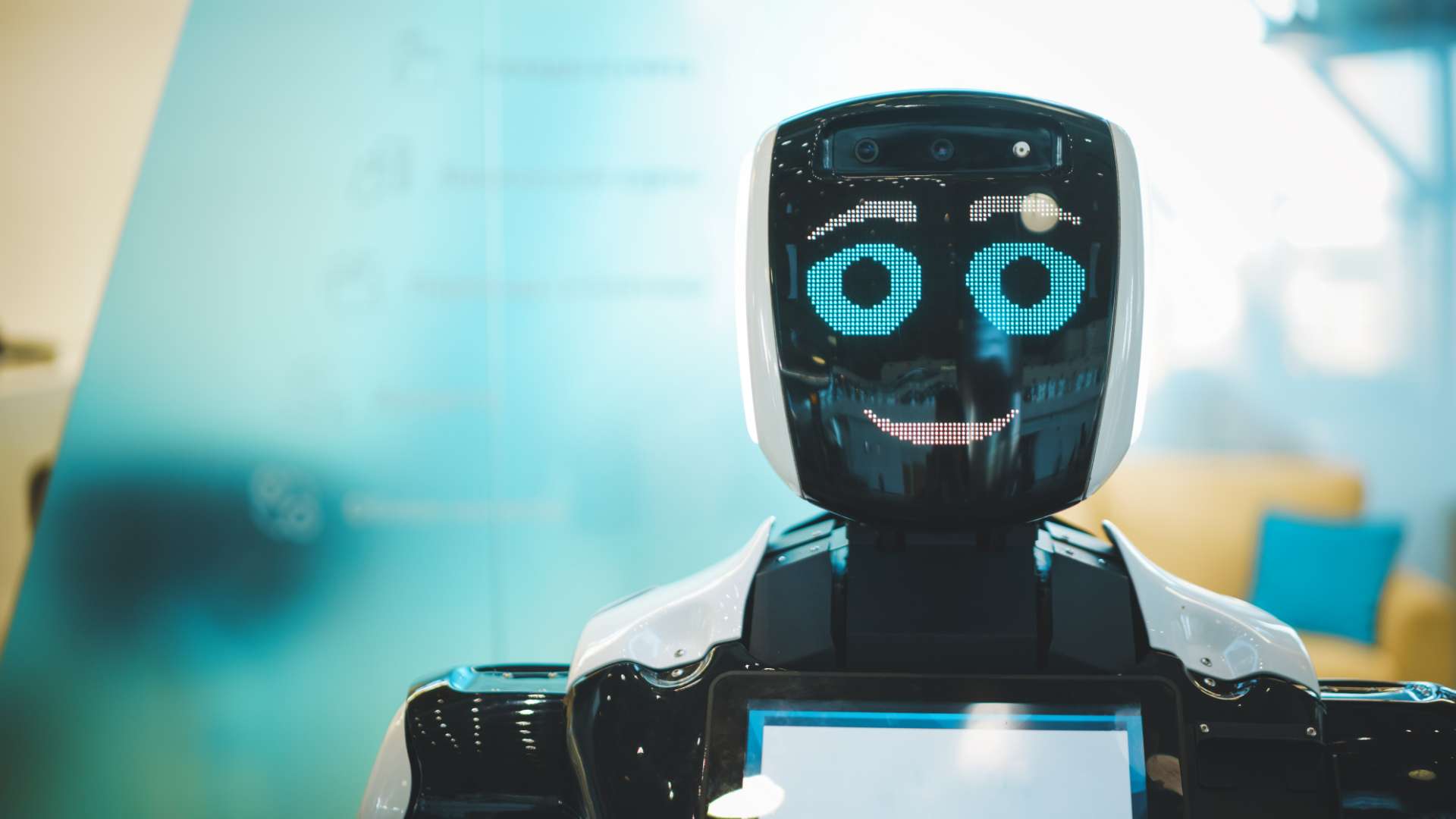
However, the hype around this new, trendy technology prevents the potential user from choosing quality solutions in a wide variety of products. This often leads to over-expectation, followed by a complete let-down. Can AI-powered video analytics really be the key to a technological breakthrough in video surveillance? We'll take a look at what the technology can do, what it can't, and where it can go from here.
Technological Breakthrough or Just Another Bubble?
It's often said that the Video Management Software (VMS) market is becoming increasingly commoditized and widely available. A lot of products with similar features (or, at least, similar promises from the manufacturer) make it hard to choose. As a result, vendor names and reputations are turning into one of their primary selling points. Manufacturers have two choices available to them: get wrapped up in a price war and rely on cutting expenses, or offer a product that's truly innovative and revolutionary.
VMS developers who choose the second route are gravitating towards creating products that use artificial intelligence based on neural networks and deep learning. Emerging two or three years ago, the AI video analytics market is experiencing a boom in growth. This new tech wave has stirred the still, stagnant backwaters of the VMS world and gave small, ambitious developers something to be optimistic about. It seems they now have a chance to emerge as market leaders in the next few years.
However, the hype around this popular trend is raising reasonable concerns among experienced security industry professionals. These concerns come from clients looking for a solution to their problems, and from suppliers building a long-term development strategy. This largely resembles another tech bubble, like the one built up around pre-AI video analytics and burst when it became clear that the sensational promises around it were pure marketing hype (and rather unscrupulously so). However, there are a lot of factors that indicate that AI-powered video surveillance systems aren't another bubble.
The first — and the main one — comes from systems already in place on customers' sites. They fulfill the same promises made during the previous bubble by hotheads in a rush to teach the computer to analyze events in real time using a classical algorithmic approach.
The second is the fact that this new technology has seen investment from not only software and cloud startups, but also established VMS developers. Even giants like Intel, which has presented a full line of neural network accelerator hardware and a set of software tools that streamlines working with them, specifically in the field of computer vision.
The third factor lies in artificial intelligence's abilities. AI plays chess, drives cars, and works wonders in many other fields. Why shouldn't it be applied to video monitoring and analysis?
What AI Can Do
Just what can artificial intelligence do in video surveillance systems at this stage of development? It can't quite analyze a sequence of events and understand the "logic" of what's happening in the cameras' field of view. At least not yet. But it's probable that AI will learn to do this in the next few years. But neural network analytics can already detect, classify, and track objects very well, providing high accuracy even in busy scenes.
Artificial intelligence can be used in the real world to:
- detect smoke and flames for early fire warning at open areas (forest, open warehouse, parking lot, etc.);
- distinguish people/vehicles from animals and other moving objects, e.g. to protect the perimeter of a nature park from poachers;
- distinguish a person in a helmet and protective clothing from a person without them to prevent accidents at a dangerous production facility or construction site;
- count objects of a specific type, e.g. cars in a parking lot, people in the sales floor, wares moving on a conveyor belt, etc. in non-security-related solutions.
Those are just a few examples. After training a neural network, it can tackle other, similar tasks, too. Generally, a neural network trained in specific conditions isn't replicable. In other words, it won't work as well under different conditions. On the other hand, developers have learned how to quickly train AI for the needs of a specific project. The most important requirement is having enough video footage.
Somewhat apart from that is the use of neural networks in facial and automatic number-plate recognition. This is an example of reproducible neural networks (train once, deploy everywhere), which makes them more appealing commercially. If non-reproducible neural networks have only recently become economically feasible due to the rapid evolution of specialized hardware (aforementioned Intel's product, for example), then the use of AI in facial recognition and ANPR has been well established for a long time.
Another kind of AI analytics that we'll explore is behavior analytics. This function, probably more than any other, is bringing video surveillance systems closer to understanding what's happening on camera. Its potential is vast.
How Behavior Analytics Works
From a technical point of view, behavior analytics combines artificial intelligence with a classic algorithmic approach. A neural network trained on a multitude of scenarios can determine the position of the bodies, heads, and limbs of humans in the camera's field of view. The algorithm outputs an array of data containing descriptions of their poses.
Conditions can be set for data to detect a specific pose, such as raised hands, prostrated or crouching persons. Developers can use this to quickly create new detection tools to identify potentially dangerous behavior specified by a government or business client. There's no need for additional training of the neural network.
How Behavior Analytics Can Be Deployed
Someone crouched down next to an ATM could be a technician, CIT guard, or burglar. Bank security should be notified in any of the cases.
A person in shooter position, together with a bank employee or cashier with their hands raised could indicate a robbery. The system can be configured to automatically send alerts with a surveillance snapshot to the police so they can assess the threat and take action if needed. It's vital that the police receive the alert, even if the employee is unable to activate the alarm.
In many cases, attention should be directed to a prostrate individual. This could be somebody who needs immediate help, or it could be someone sleeping in an inappropriate public place, for example, a 24/7 ATM space.
Behavioral analytics can also be used to ensure workplace safety. For example, tracking whether employees are holding the handrails when using the stairs at a manufacturing facility or a construction site.
What Now?
Behavior analytics can be deployed wherever your clients' imagination takes them. With this feature, practically any pose that indicates potentially dangerous behavior can be detected. Timely response to an alarm helps avoid material damages or, in other situations, casualties.
An area of potential development for behavior analytics is the ability to analyze a sequence of poses by the same person or a combination of poses and relative positions of several individuals. That will be the next level of evolution in AI's use in video surveillance: moving from "detecting" to "understanding" behavior in real time.
In its most basic form, this type of analytics can be deployed to detect deviations from the search procedure in correctional facilities when a person being inspected must assume a pre-defined sequence of poses. A more advanced form allows it to detect any kind of abnormal behavior, such as a brawl breaking out in a public space. Ideally, behavior analytics can predict dangerous situations based on nearly imperceptible cues gleaned from collected statistics and a Big Data analysis.
At the moment, this sounds like pure fantasy, but what seemed like whimsy not too long ago is now a reality with AI. It's already beaten humans in chess and the game of Go (Weiqi). Will artificial intelligence be able to outplay humans at charades one day? It's entirely possible that we'll soon see for ourselves.
This article was originally published on securityinformed.com
블로그로 돌아가기